I saw this project and was immediately impressed with the ingenuity and engineering that went into it. So naturally it got me thinking about what the next steps would be. I'm wondering if we can't fine tune the rewards to minimize play time and try and beat the speedruns. I'm thinking that by feeding additional memory poked data like map ids, enemy pokemon, badge times, etc in addition to the frame buffer as input features as well as generating higher rewards based on milestone times, we can start seeing some competitive runs out of Roko's Basilisk here.
I'll be trying stuff out in my spare time.
as of right now I'm only pushing code changes and not checkpoints since I'm coding on a separate machine...so the pretrained model provided will not include speedrun features or rewards.
Original README below:

Watch the Video on Youtube!
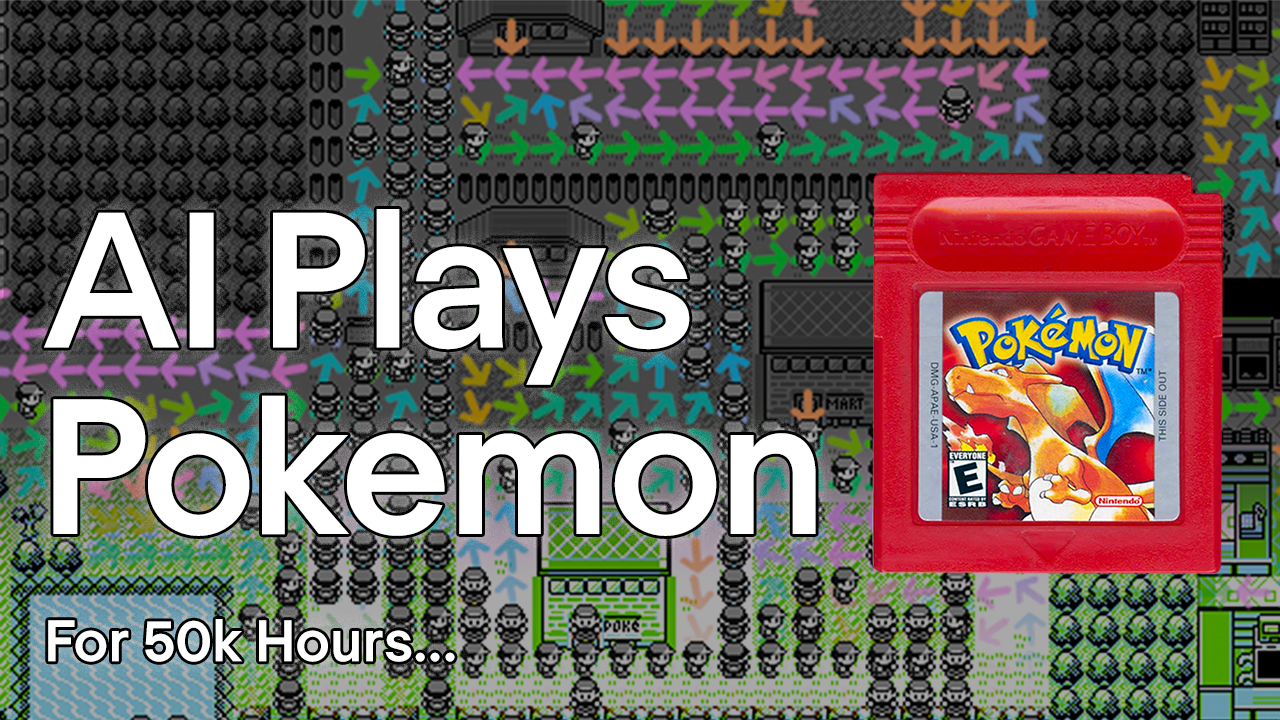
🐍 Python 3.10 is recommended. Other versions may work but have not been tested.
- Copy your legally obtained Pokemon Red ROM into the base directory. You can find this using google, it should be 1MB. Rename it to
PokemonRed.gb
if it is not already. The sha1 sum should beea9bcae617fdf159b045185467ae58b2e4a48b9a
, which you can verify by runningshasum PokemonRed.gb
. - Move into the
baselines/
directory:
cd baselines
- Install dependencies:
pip install -r requirements.txt
It may be necessary in some cases to separately install the SDL libraries. - Run:
python run_pretrained_interactive.py
By default the game with terminate after 32K steps, or ~1 hour. You can increase this by adjusting the ep_length
variable, but it will also use more memory.
Interact with the emulator using the arrow keys and the a
and s
keys (A and B buttons).
You can pause the AI's input during the game by editing agent_enabled.txt
Note: the Pokemon.gb file MUST be in the main directory and your current directory MUST be the baselines/
directory in order for this to work.
Note: By default this can use up to ~100G of RAM. You can decrease this by reducing the num_cpu
or ep_length
, but it may affect the results. Also, the model behavior may become degenerate for up to the first 50 training iterations or so before starting to improve. This could likely be fixed with better hyperparameters but I haven't had the time or resources to sweep these.
- Previous steps 1-3
- Run:
python run_baseline_parallel.py
You can view the current state of each emulator, plot basic stats, and compare to previous runs using the VisualizeProgress.ipynb
notebook.
Map visualization code can be found in visualization/
directory.