forked from trailbehind/DeepOSM
-
Notifications
You must be signed in to change notification settings - Fork 0
Commit
This commit does not belong to any branch on this repository, and may belong to a fork outside of the repository.
detect mis-registered OSM roads (by default in Delaware) (trailbehind#46
) * fix odd issue with tflearn/pil imports * fix radnomization issue too * undo test line changes * fix flake complaint * add a method to bundle of image, label, and location data for the UI/database * didnt need that dockerfile change * pep cetera * code to enumerate false negatives and false positives for all naip tiles * this seems to make list properly now, let's runn it on gpu * running this on gpu with an 80% accurate net to see how many false pos/negs that generates in all 170+ delaware NAIPs * typo * pep * more pep * use normal minibatching technique * add terrapattern to README * switch computers * correct false-pos/neg loops * pep8 * set defaults that do the delaware false positive analysis quickly as possible * update README to say what the script does by default * typos and such in README * fix bug from line spacing * add development seed's project to the readme
- Loading branch information
1 parent
3d6aba9
commit 92983f0
Showing
6 changed files
with
156 additions
and
61 deletions.
There are no files selected for viewing
This file contains bidirectional Unicode text that may be interpreted or compiled differently than what appears below. To review, open the file in an editor that reveals hidden Unicode characters.
Learn more about bidirectional Unicode characters
Original file line number | Diff line number | Diff line change |
---|---|---|
@@ -1,37 +1,39 @@ | ||
# DeepOSM | ||
|
||
Detect roads and features in satellite imagery, by training neural networks with OpenStreetMap (OSM) data. This code lets you: | ||
Classify roads and features in satellite imagery, by training neural networks with OpenStreetMap (OSM) data. DeepOSM lets you: | ||
|
||
* Download a chunk of satellite imagery | ||
* Download OSM data that shows roads/features for that area | ||
* Generate training and evaluation data | ||
* Display predictions of mis-registered roads in OSM data, or display raw predictions of ON/OFF | ||
|
||
Running the code is as easy as install Docker, make dev, and run a script. | ||
|
||
Contributions are welcome. Open an issue if you want to discuss something to do, or [email me](mailto:[email protected]). | ||
|
||
## Default Data/Accuracy | ||
|
||
By default, DeepOSM will download the minimum necessary training data, and use the simplest possible network. | ||
By default, DeepOSM will analyze about 200 sq. km of area in Delaware. DeepOSM will | ||
|
||
* It will predict if the center 9px of a 64px tile contains road. | ||
* It will only use the infrared (IR) band, not the RGB bands. | ||
* It will be about 65% accurate, based on how the training/test data is constructed. | ||
* It will use a single fully connected relu layer in [TensorFlow](https://www.tensorflow.org/). | ||
* predict if the center 9px of a 64px tile contains road. | ||
* use the infrared (IR) band and RGB bands. | ||
* be 75-80% accurate overall, training only for a minute or so. | ||
* use a single fully-connected relu layer in [TensorFlow](https://www.tensorflow.org/). | ||
* render, as JPEGs, "false positive" predictions in the OSM data - i.e. where OSM lists a road, but DeepOSM thinks there isn't one. | ||
|
||
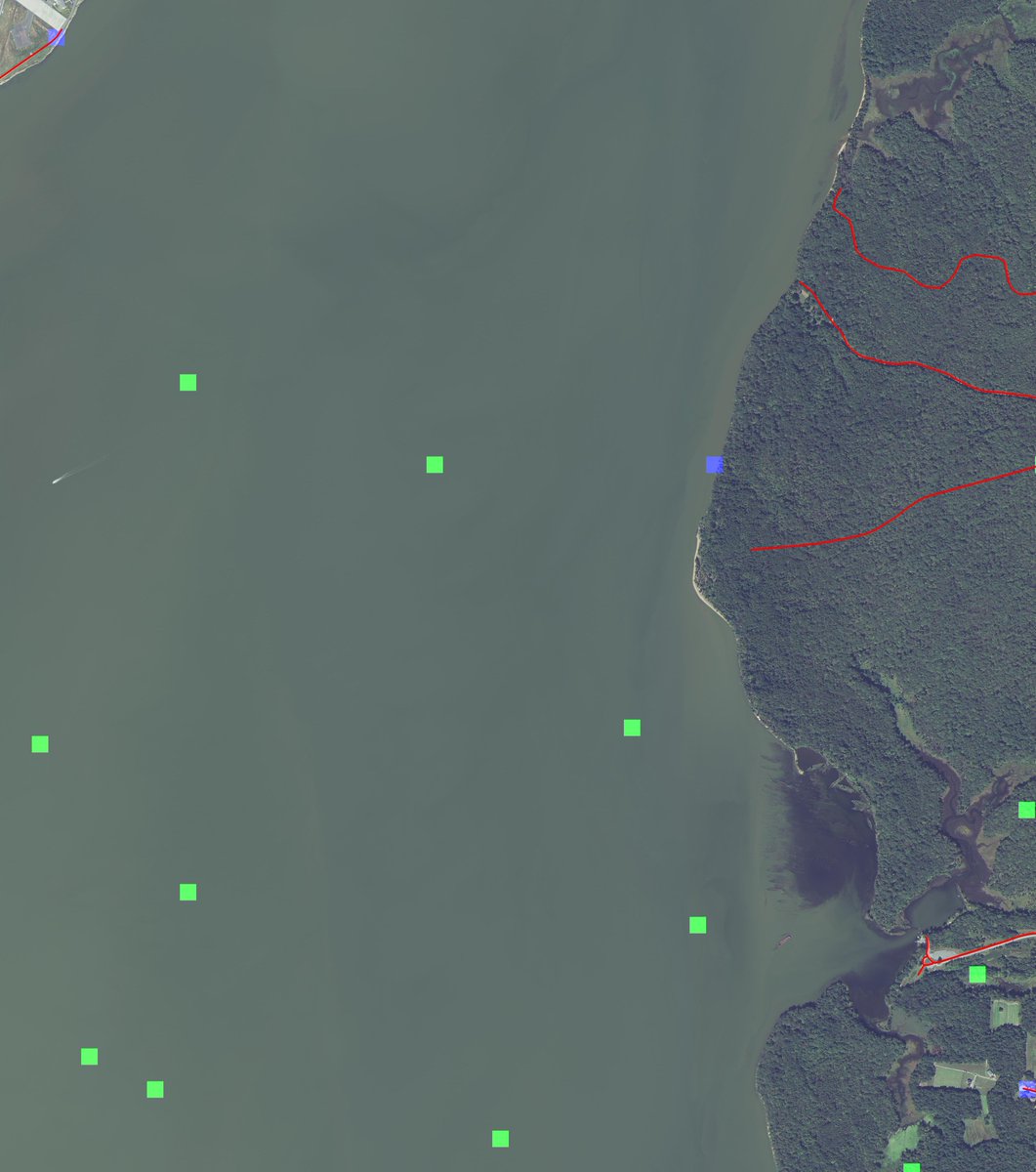 | ||
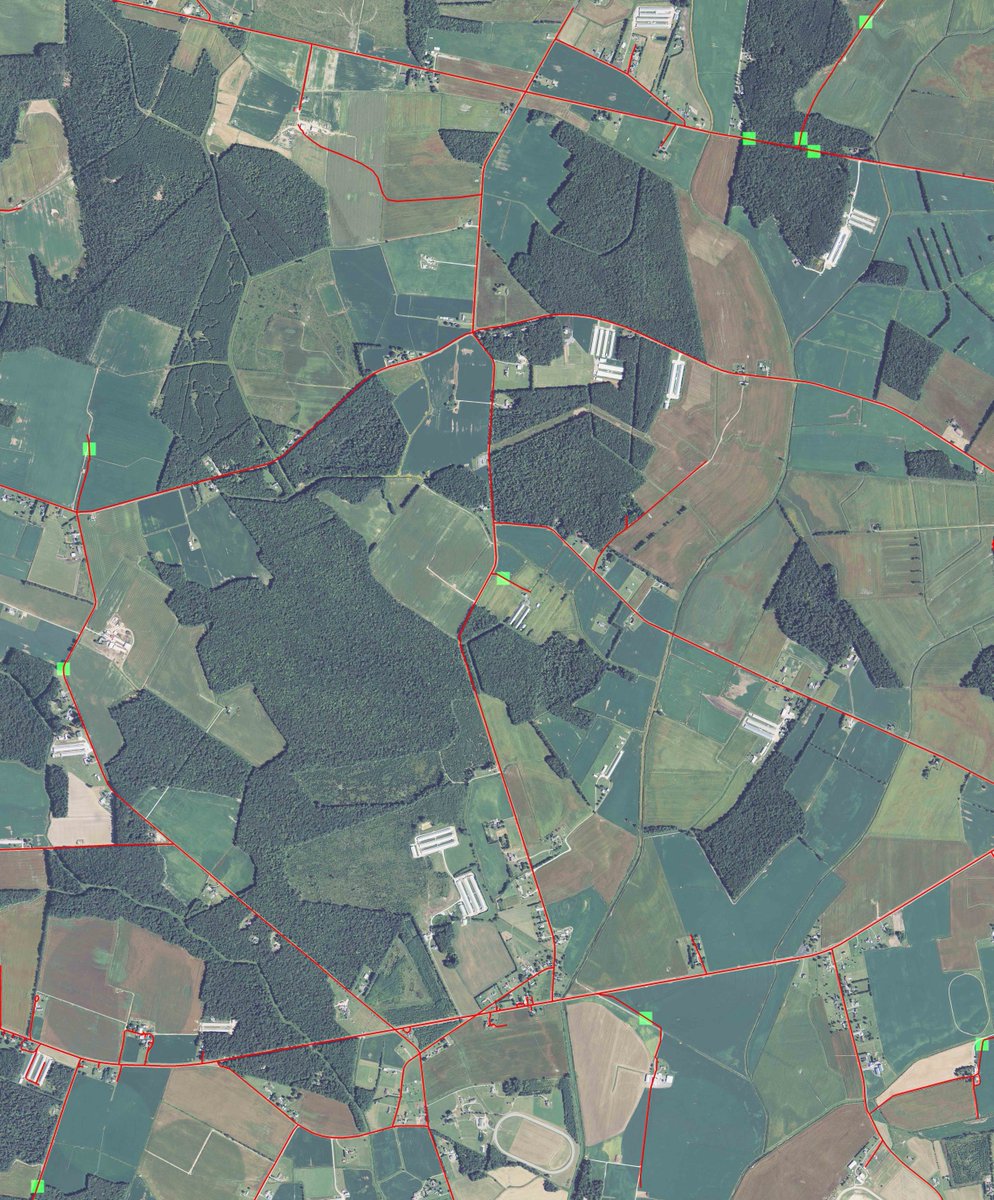 | ||
|
||
## Background on Data - NAIPs and OSM PBF | ||
|
||
For training data, DeepOSM cuts tiles out of [NAIP images](http://www.fsa.usda.gov/programs-and-services/aerial-photography/imagery-programs/naip-imagery/), which provide 1 meter per pixel resolution, with RGB+infrared data bands. | ||
For training data, DeepOSM cuts tiles out of [NAIP images](http://www.fsa.usda.gov/programs-and-services/aerial-photography/imagery-programs/naip-imagery/), which provide 1-meter-per-pixel resolution, with RGB+infrared data bands. | ||
|
||
For training labels, DeepOSM uses PBF extracts of OSM data, which contain features/ways in binary format, which can be munged with Python. | ||
For training labels, DeepOSM uses PBF extracts of OSM data, which contain features/ways in binary format that can be munged with Python. | ||
|
||
The [NAIPs come from a requester pays bucket on S3 set up by Mapbox](http://www.slideshare.net/AmazonWebServices/open-data-innovation-building-on-open-data-sets-for-innovative-applications), and the OSM extracts come [from geofabrik](http://download.geofabrik.de/). | ||
|
||
## Install Requirements | ||
|
||
DeepOSM has been run successfully on both Mac and Linux (14.04 and 16.04). You need at least 4GB of memory. | ||
DeepOSM has been run successfully on both Mac (10.x) and Linux (14.04 and 16.04). You need at least 4GB of memory. | ||
|
||
### AWS Credentials | ||
|
||
|
@@ -68,14 +70,14 @@ make dev | |
|
||
Inside Docker, the following Python scripts will work. This will download all source data, tile it into training/test data and labels, train the neural net, and generate image and text output. | ||
|
||
The default data is eight NAIPs, which gets tiled into NxNx4 bands of data (RGB-IR bands). The training labels derive from PBF files that overlap the NAIPs. | ||
The default data is six NAIPs, which get tiled into 64x64x4 bands of data (RGB-IR bands). The training labels derive from PBF files that overlap the NAIPs. | ||
|
||
``` | ||
python bin/create_training_data.py | ||
python bin/train_neural_net.py | ||
``` | ||
|
||
For output, it will produce some console logs, and then JPEGs of the ways, labels, and predictions overlaid on the tiff. | ||
For output, DeepOSM will produce some console logs, and then JPEGs of the ways, labels, and predictions overlaid on the tiff. | ||
|
||
### Jupyter Notebook | ||
|
||
|
@@ -105,38 +107,41 @@ Images](http://citeseerx.ist.psu.edu/viewdoc/download?doi=10.1.1.232.1679&rep=re | |
best/recent paper on doing this, great success with these methods | ||
* Similar Efforts with OSM Data | ||
* [OSM-Crosswalk-Detection](https://github.com/geometalab/OSM-Crosswalk-Detection) - uses Keras to detect crosswalks, a class project (Fall 2015) | ||
* [OSM-HOT-ConvNet](https://github.com/larsroemheld/OSM-HOT-ConvNet) - attempted use for disaster response, author thinks it's only 69% accurate at pixel level (Fall 2016) | ||
* [OSM-HOT-ConvNet](https://github.com/larsroemheld/OSM-HOT-ConvNet) - attempted use for disaster response, author thinks it's only 69% accurate at pixel level (fall 2016) | ||
* [Terrapattern](http://www.terrapattern.com/) - (spring 2016) - detect similar images, such as pools, boat wakes, or other patterns journalists/researchers might be interested in - Carnegie Mellon researchers, open source | ||
* [Skynet Data](https://github.com/developmentseed/skynet-data) - (spring 2016) - data pipeline for machine learning with OpenStreetMap | ||
|
||
|
||
* [Parsing Natural Scenes and Natural Language | ||
with Recursive Neural Networks (RNNs)](http://ai.stanford.edu/~ang/papers/icml11-ParsingWithRecursiveNeuralNetworks.pdf) | ||
* Links from the Tensorflow site | ||
* Background on Neural Networks and Deep Learning | ||
* [MNIST Data and Background](http://yann.lecun.com/exdb/mnist/) | ||
* all the other links to Nielsen’s book and [Colah’s blog](http://colah.github.io/posts/2015-08-Backprop/) | ||
* Deep Background | ||
* [original Information Theory paper by Shannon](http://worrydream.com/refs/Shannon%20-%20A%20Mathematical%20Theory%20of%20Communication.pdf) | ||
|
||
[Also see a work journal here](http://trailbehind.github.io/DeepOSM/). | ||
|
||
### Papers - Relevant Maybe | ||
|
||
* [Uses a large window to improve predictions, trying to capture broad network topology](https://www.inf.ethz.ch/personal/ladickyl/roads_gcpr14.pdf) | ||
* [Uses a large window to improve predictions, trying to capture broad network topology.](https://www.inf.ethz.ch/personal/ladickyl/roads_gcpr14.pdf) | ||
|
||
* [Automatically extract roads with no human labels. Not that accurate, could work for preprocessing to detect roads in unlab](https://www.researchgate.net/publication/263892800_Tensor-Cuts_A_simultaneous_multi-type_feature_extractor_and_classifier_and_its_application_to_road_extraction_from_satellite_images) | ||
* [Automatically extract roads with no human labels. Not that accurate, could work for preprocessing to detect roads.](https://www.researchgate.net/publication/263892800_Tensor-Cuts_A_simultaneous_multi-type_feature_extractor_and_classifier_and_its_application_to_road_extraction_from_satellite_images) | ||
|
||
### Papers - Not All that Relevant | ||
|
||
* [Uses map data and shapes of overpasses to then detect pictures of the objects? Seems like a cool paper to read if it was free.](http://dl.acm.org/citation.cfm?id=2424336) | ||
|
||
* [New technique for classification of sub-half-meter data into different zones](http://ieeexplore.ieee.org/xpl/login.jsp?tp=&arnumber=6827949&url=http%3A%2F%2Fieeexplore.ieee.org%2Fxpls%2Fabs_all.jsp%3Farnumber%3D6827949) | ||
* [New technique for classification of sub-half-meter data into different zones.](http://ieeexplore.ieee.org/xpl/login.jsp?tp=&arnumber=6827949&url=http%3A%2F%2Fieeexplore.ieee.org%2Fxpls%2Fabs_all.jsp%3Farnumber%3D6827949) | ||
|
||
* [Couldn't access text, focused on usig lidar data](http://ieeexplore.ieee.org/xpl/login.jsp?tp=&arnumber=6238909&url=http%3A%2F%2Fieeexplore.ieee.org%2Fxpls%2Fabs_all.jsp%3Farnumber%3D6238909) | ||
* [Couldn't access text, focused on using lidar data.](http://ieeexplore.ieee.org/xpl/login.jsp?tp=&arnumber=6238909&url=http%3A%2F%2Fieeexplore.ieee.org%2Fxpls%2Fabs_all.jsp%3Farnumber%3D6238909) | ||
|
||
* [Proposes a way to extract network topology, and maybe this can be used as a post processor?](http://www.cv-foundation.org/openaccess/content_cvpr_2013/html/Wegner_A_Higher-Order_CRF_2013_CVPR_paper.html) | ||
|
||
### Papers to Review | ||
### Papers to Review | ||
|
||
Recent Recommendations | ||
|
||
* FIND - have you seen a paper from a few years ago about estimating osm completeness by comparing size of compressed satellite images vs number of osm nodes | ||
* FIND - have you seen a paper from a few years ago about estimating OSM completeness by comparing size of compressed satellite images vs number of osm nodes | ||
|
||
* READ - this presentation on using GPS traces to suggest OSM edits (Strava/Telenav): http://webcache.googleusercontent.com/search?q=cache:VoiCwRHOyLUJ:stateofthemap.us/map-tracing-for-millennials/+&cd=3&hl=en&ct=clnk&gl=us | ||
|
||
|
This file contains bidirectional Unicode text that may be interpreted or compiled differently than what appears below. To review, open the file in an editor that reveals hidden Unicode characters.
Learn more about bidirectional Unicode characters
This file contains bidirectional Unicode text that may be interpreted or compiled differently than what appears below. To review, open the file in an editor that reveals hidden Unicode characters.
Learn more about bidirectional Unicode characters
Oops, something went wrong.