forked from huggingface/diffusers
-
Notifications
You must be signed in to change notification settings - Fork 0
Commit
This commit does not belong to any branch on this repository, and may belong to a fork outside of the repository.
* Quick implementation of t2i-adapter Load adapter module with from_pretrained Prototyping generalized adapter framework Writeup doc string for sideload framework(WIP) + some minor update on implementation Update adapter models Remove old adapter optional args in UNet Add StableDiffusionAdapterPipeline unit test Handle cpu offload in StableDiffusionAdapterPipeline Auto correct coding style Update model repo name to "RzZ/sd-v1-4-adapter-pipeline" Refactor MultiAdapter to better compatible with config system Export MultiAdapter Create pipeline document template from controlnet Create dummy objects Supproting new AdapterLight model Fix StableDiffusionAdapterPipeline common pipeline test [WIP] Update adapter pipeline document Handle num_inference_steps in StableDiffusionAdapterPipeline Update definition of Adapter "channels_in" Update documents Apply code style Fix doc typo and merge error Update doc string and example Quality of life improvement Remove redundant code and file from prototyping Remove unused pageage Remove comments Fix title Fix typo Add conditioning scale arg Bring back old implmentation Offload sideload Add supply info on document Update src/diffusers/models/adapter.py Co-authored-by: Will Berman <[email protected]> Update MultiAdapter constructor Swap out custom checkpoint and update pipeline constructor Update docment Apply suggestions from code review Co-authored-by: Will Berman <[email protected]> Correcting style Following single-file policy Update auto size in image preprocess func Update src/diffusers/pipelines/stable_diffusion/pipeline_stable_diffusion_adapter.py Co-authored-by: Will Berman <[email protected]> fix copies Update adapter pipeline behavior Add adapter_conditioning_scale doc string Add the missing doc string Apply suggestions from code review Co-authored-by: Patrick von Platen <[email protected]> Fix few bugs from suggestion Handle L-mode PIL image as control image Rename to differentiate adapter resblock Update src/diffusers/models/adapter.py Co-authored-by: Sayak Paul <[email protected]> Fix typo Update adapter parameter name Update test case and code style Fix copies Fix typo Update src/diffusers/pipelines/stable_diffusion/pipeline_stable_diffusion_adapter.py Co-authored-by: Will Berman <[email protected]> Update Adapter class name Add checkpoint converting script Fix style Fix-copies Remove dev script Apply suggestions from code review Co-authored-by: Patrick von Platen <[email protected]> Updates for parameter rename Fix convert_adapter remove main fix diff more refactoring more more small fixes refactor tests more slow tests more tests Update docs/source/en/api/pipelines/overview.mdx Co-authored-by: Sayak Paul <[email protected]> add community contributor to docs Update docs/source/en/api/pipelines/stable_diffusion/adapter.mdx Co-authored-by: Sayak Paul <[email protected]> Update docs/source/en/api/pipelines/stable_diffusion/adapter.mdx Co-authored-by: Sayak Paul <[email protected]> Update docs/source/en/api/pipelines/stable_diffusion/adapter.mdx Co-authored-by: Sayak Paul <[email protected]> Update docs/source/en/api/pipelines/stable_diffusion/adapter.mdx Co-authored-by: Sayak Paul <[email protected]> Update docs/source/en/api/pipelines/stable_diffusion/adapter.mdx Co-authored-by: Sayak Paul <[email protected]> fix remove from_adapters license paper link docs more url fixes more docs fix fixes fix fix * fix sample inplace add * additional_kwargs -> additional_residuals * move t2i adapter pipeline to own module * preprocess -> _preprocess_adapter_image * add TencentArc to license * fix example code links * add image converter and fix example doc string * fix links * clearer additional residual application --------- Co-authored-by: HimariO <[email protected]>
- Loading branch information
1 parent
3929954
commit a0597f3
Showing
18 changed files
with
1,989 additions
and
9 deletions.
There are no files selected for viewing
This file contains bidirectional Unicode text that may be interpreted or compiled differently than what appears below. To review, open the file in an editor that reveals hidden Unicode characters.
Learn more about bidirectional Unicode characters
This file contains bidirectional Unicode text that may be interpreted or compiled differently than what appears below. To review, open the file in an editor that reveals hidden Unicode characters.
Learn more about bidirectional Unicode characters
187 changes: 187 additions & 0 deletions
187
docs/source/en/api/pipelines/stable_diffusion/adapter.mdx
This file contains bidirectional Unicode text that may be interpreted or compiled differently than what appears below. To review, open the file in an editor that reveals hidden Unicode characters.
Learn more about bidirectional Unicode characters
Original file line number | Diff line number | Diff line change |
---|---|---|
@@ -0,0 +1,187 @@ | ||
<!--Copyright 2023 The HuggingFace Team. All rights reserved. | ||
|
||
Licensed under the Apache License, Version 2.0 (the "License"); you may not use this file except in compliance with | ||
the License. You may obtain a copy of the License at | ||
|
||
http://www.apache.org/licenses/LICENSE-2.0 | ||
|
||
Unless required by applicable law or agreed to in writing, software distributed under the License is distributed on | ||
an "AS IS" BASIS, WITHOUT WARRANTIES OR CONDITIONS OF ANY KIND, either express or implied. See the License for the | ||
specific language governing permissions and limitations under the License. | ||
--> | ||
|
||
# Text-to-Image Generation with Adapter Conditioning | ||
|
||
## Overview | ||
|
||
[T2I-Adapter: Learning Adapters to Dig out More Controllable Ability for Text-to-Image Diffusion Models](https://arxiv.org/abs/2302.08453) by Chong Mou, Xintao Wang, Liangbin Xie, Jian Zhang, Zhongang Qi, Ying Shan, Xiaohu Qie. | ||
|
||
Using the pretrained models we can provide control images (for example, a depth map) to control Stable Diffusion text-to-image generation so that it follows the structure of the depth image and fills in the details. | ||
|
||
The abstract of the paper is the following: | ||
|
||
*The incredible generative ability of large-scale text-to-image (T2I) models has demonstrated strong power of learning complex structures and meaningful semantics. However, relying solely on text prompts cannot fully take advantage of the knowledge learned by the model, especially when flexible and accurate structure control is needed. In this paper, we aim to ``dig out" the capabilities that T2I models have implicitly learned, and then explicitly use them to control the generation more granularly. Specifically, we propose to learn simple and small T2I-Adapters to align internal knowledge in T2I models with external control signals, while freezing the original large T2I models. In this way, we can train various adapters according to different conditions, and achieve rich control and editing effects. Further, the proposed T2I-Adapters have attractive properties of practical value, such as composability and generalization ability. Extensive experiments demonstrate that our T2I-Adapter has promising generation quality and a wide range of applications.* | ||
|
||
This model was contributed by the community contributor [HimariO](https://github.com/HimariO) ❤️ . | ||
|
||
## Available Pipelines: | ||
|
||
| Pipeline | Tasks | Demo | ||
|---|---|:---:| | ||
| [StableDiffusionAdapterPipeline](https://github.com/huggingface/diffusers/blob/main/src/diffusers/pipelines/stable_diffusion/pipeline_stable_diffusion_adapter.py) | *Text-to-Image Generation with T2I-Adapter Conditioning* | - | ||
|
||
## Usage example | ||
|
||
In the following we give a simple example of how to use a *T2IAdapter* checkpoint with Diffusers for inference. | ||
All adapters use the same pipeline. | ||
|
||
1. Images are first converted into the appropriate *control image* format. | ||
2. The *control image* and *prompt* are passed to the [`StableDiffusionAdapterPipeline`]. | ||
|
||
Let's have a look at a simple example using the [Color Adapter](https://huggingface.co/TencentARC/t2iadapter_color_sd14v1). | ||
|
||
```python | ||
from diffusers.utils import load_image | ||
|
||
image = load_image("https://huggingface.co/datasets/diffusers/docs-images/resolve/main/t2i-adapter/color_ref.png") | ||
``` | ||
|
||
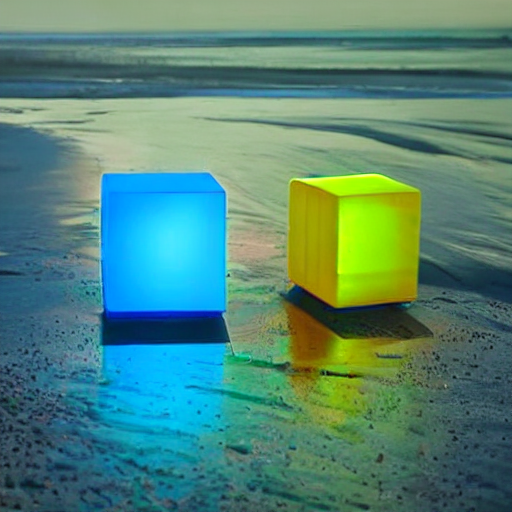 | ||
|
||
|
||
Then we can create our color palette by simply resizing it to 8 by 8 pixels and then scaling it back to original size. | ||
|
||
```python | ||
from PIL import Image | ||
|
||
color_palette = image.resize((8, 8)) | ||
color_palette = color_palette.resize((512, 512), resample=Image.Resampling.NEAREST) | ||
``` | ||
|
||
Let's take a look at the processed image. | ||
|
||
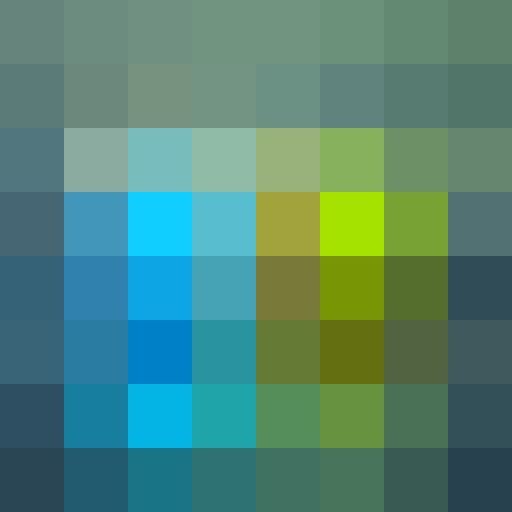 | ||
|
||
|
||
Next, create the adapter pipeline | ||
|
||
```py | ||
import torch | ||
from diffusers import StableDiffusionAdapterPipeline, T2IAdapter | ||
|
||
adapter = T2IAdapter.from_pretrained("TencentARC/t2iadapter_color_sd14v1") | ||
pipe = StableDiffusionAdapterPipeline.from_pretrained( | ||
"CompVis/stable-diffusion-v1-4", | ||
adapter=adapter, | ||
torch_dtype=torch.float16, | ||
) | ||
pipe.to("cuda") | ||
``` | ||
|
||
Finally, pass the prompt and control image to the pipeline | ||
|
||
```py | ||
# fix the random seed, so you will get the same result as the example | ||
generator = torch.manual_seed(7) | ||
|
||
out_image = pipe( | ||
"At night, glowing cubes in front of the beach", | ||
image=color_palette, | ||
generator=generator, | ||
).images[0] | ||
``` | ||
|
||
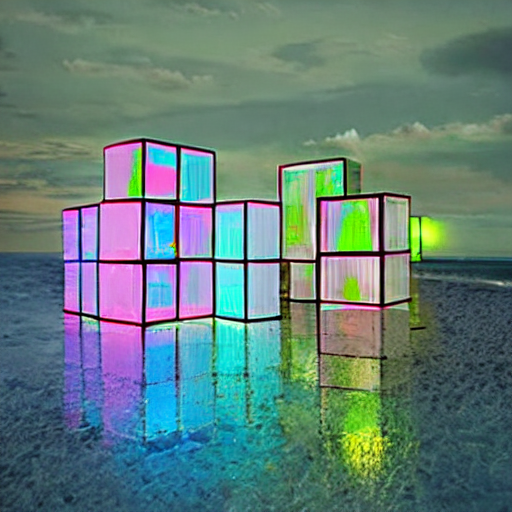 | ||
|
||
|
||
## Available checkpoints | ||
|
||
Non-diffusers checkpoints can be found under [TencentARC/T2I-Adapter](https://huggingface.co/TencentARC/T2I-Adapter/tree/main/models). | ||
|
||
### T2I-Adapter with Stable Diffusion 1.4 | ||
|
||
| Model Name | Control Image Overview| Control Image Example | Generated Image Example | | ||
|---|---|---|---| | ||
|[TencentARC/t2iadapter_color_sd14v1](https://huggingface.co/TencentARC/t2iadapter_color_sd14v1)<br/> *Trained with spatial color palette* | A image with 8x8 color palette.|<a href="https://huggingface.co/datasets/diffusers/docs-images/resolve/main/t2i-adapter/color_sample_input.png"><img width="64" style="margin:0;padding:0;" src="https://huggingface.co/datasets/diffusers/docs-images/resolve/main/t2i-adapter/color_sample_input.png"/></a>|<a href="https://huggingface.co/datasets/diffusers/docs-images/resolve/main/t2i-adapter/color_sample_output.png"><img width="64" src="https://huggingface.co/datasets/diffusers/docs-images/resolve/main/t2i-adapter/color_sample_output.png"/></a>| | ||
|[TencentARC/t2iadapter_canny_sd14v1](https://huggingface.co/TencentARC/t2iadapter_canny_sd14v1)<br/> *Trained with canny edge detection* | A monochrome image with white edges on a black background.|<a href="https://huggingface.co/datasets/diffusers/docs-images/resolve/main/t2i-adapter/canny_sample_input.png"><img width="64" style="margin:0;padding:0;" src="https://huggingface.co/datasets/diffusers/docs-images/resolve/main/t2i-adapter/canny_sample_input.png"/></a>|<a href="https://huggingface.co/datasets/diffusers/docs-images/resolve/main/t2i-adapter/canny_sample_output.png"><img width="64" src="https://huggingface.co/datasets/diffusers/docs-images/resolve/main/t2i-adapter/canny_sample_output.png"/></a>| | ||
|[TencentARC/t2iadapter_sketch_sd14v1](https://huggingface.co/TencentARC/t2iadapter_sketch_sd14v1)<br/> *Trained with [PidiNet](https://github.com/zhuoinoulu/pidinet) edge detection* | A hand-drawn monochrome image with white outlines on a black background.|<a href="https://huggingface.co/datasets/diffusers/docs-images/resolve/main/t2i-adapter/sketch_sample_input.png"><img width="64" style="margin:0;padding:0;" src="https://huggingface.co/datasets/diffusers/docs-images/resolve/main/t2i-adapter/sketch_sample_input.png"/></a>|<a href="https://huggingface.co/datasets/diffusers/docs-images/resolve/main/t2i-adapter/sketch_sample_output.png"><img width="64" src="https://huggingface.co/datasets/diffusers/docs-images/resolve/main/t2i-adapter/sketch_sample_output.png"/></a>| | ||
|[TencentARC/t2iadapter_depth_sd14v1](https://huggingface.co/TencentARC/t2iadapter_depth_sd14v1)<br/> *Trained with Midas depth estimation* | A grayscale image with black representing deep areas and white representing shallow areas.|<a href="https://huggingface.co/datasets/diffusers/docs-images/resolve/main/t2i-adapter/depth_sample_input.png"><img width="64" src="https://huggingface.co/datasets/diffusers/docs-images/resolve/main/t2i-adapter/depth_sample_input.png"/></a>|<a href="https://huggingface.co/datasets/diffusers/docs-images/resolve/main/t2i-adapter/depth_sample_output.png"><img width="64" src="https://huggingface.co/datasets/diffusers/docs-images/resolve/main/t2i-adapter/depth_sample_output.png"/></a>| | ||
|[TencentARC/t2iadapter_openpose_sd14v1](https://huggingface.co/TencentARC/t2iadapter_openpose_sd14v1)<br/> *Trained with OpenPose bone image* | A [OpenPose bone](https://github.com/CMU-Perceptual-Computing-Lab/openpose) image.|<a href="https://huggingface.co/datasets/diffusers/docs-images/resolve/main/t2i-adapter/openpose_sample_input.png"><img width="64" src="https://huggingface.co/datasets/diffusers/docs-images/resolve/main/t2i-adapter/openpose_sample_input.png"/></a>|<a href="https://huggingface.co/datasets/diffusers/docs-images/resolve/main/t2i-adapter/openpose_sample_output.png"><img width="64" src="https://huggingface.co/datasets/diffusers/docs-images/resolve/main/t2i-adapter/openpose_sample_output.png"/></a>| | ||
|[TencentARC/t2iadapter_keypose_sd14v1](https://huggingface.co/TencentARC/t2iadapter_keypose_sd14v1)<br/> *Trained with mmpose skeleton image* | A [mmpose skeleton](https://github.com/open-mmlab/mmpose) image.|<a href="https://huggingface.co/datasets/diffusers/docs-images/resolve/main/t2i-adapter/keypose_sample_input.png"><img width="64" src="https://huggingface.co/datasets/diffusers/docs-images/resolve/main/t2i-adapter/keypose_sample_input.png"/></a>|<a href="https://huggingface.co/datasets/diffusers/docs-images/resolve/main/t2i-adapter/keypose_sample_output.png"><img width="64" src="https://huggingface.co/datasets/diffusers/docs-images/resolve/main/t2i-adapter/keypose_sample_output.png"/></a>| | ||
|[TencentARC/t2iadapter_seg_sd14v1](https://huggingface.co/TencentARC/t2iadapter_seg_sd14v1)<br/>*Trained with semantic segmentation* | An [custom](https://github.com/TencentARC/T2I-Adapter/discussions/25) segmentation protocol image.|<a href="https://huggingface.co/datasets/diffusers/docs-images/resolve/main/t2i-adapter/seg_sample_input.png"><img width="64" src="https://huggingface.co/datasets/diffusers/docs-images/resolve/main/t2i-adapter/seg_sample_input.png"/></a>|<a href="https://huggingface.co/datasets/diffusers/docs-images/resolve/main/t2i-adapter/seg_sample_output.png"><img width="64" src="https://huggingface.co/datasets/diffusers/docs-images/resolve/main/t2i-adapter/seg_sample_output.png"/></a> | | ||
|[TencentARC/t2iadapter_canny_sd15v2](https://huggingface.co/TencentARC/t2iadapter_canny_sd15v2)|| | ||
|[TencentARC/t2iadapter_depth_sd15v2](https://huggingface.co/TencentARC/t2iadapter_depth_sd15v2)|| | ||
|[TencentARC/t2iadapter_sketch_sd15v2](https://huggingface.co/TencentARC/t2iadapter_sketch_sd15v2)|| | ||
|[TencentARC/t2iadapter_zoedepth_sd15v1](https://huggingface.co/TencentARC/t2iadapter_zoedepth_sd15v1)|| | ||
|
||
## Combining multiple adapters | ||
|
||
[`MultiAdapter`] can be used for applying multiple conditionings at once. | ||
|
||
Here we use the keypose adapter for the character posture and the depth adapter for creating the scene. | ||
|
||
```py | ||
import torch | ||
from PIL import Image | ||
from diffusers.utils import load_image | ||
|
||
cond_keypose = load_image( | ||
"https://huggingface.co/datasets/diffusers/docs-images/resolve/main/t2i-adapter/keypose_sample_input.png" | ||
) | ||
cond_depth = load_image( | ||
"https://huggingface.co/datasets/diffusers/docs-images/resolve/main/t2i-adapter/depth_sample_input.png" | ||
) | ||
cond = [[cond_keypose, cond_depth]] | ||
|
||
prompt = ["A man walking in an office room with a nice view"] | ||
``` | ||
|
||
The two control images look as such: | ||
|
||
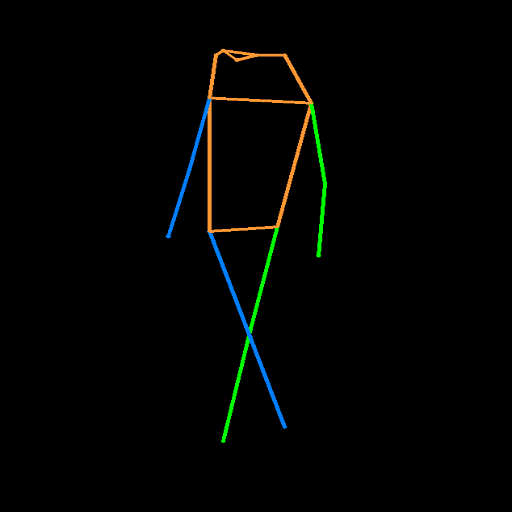 | ||
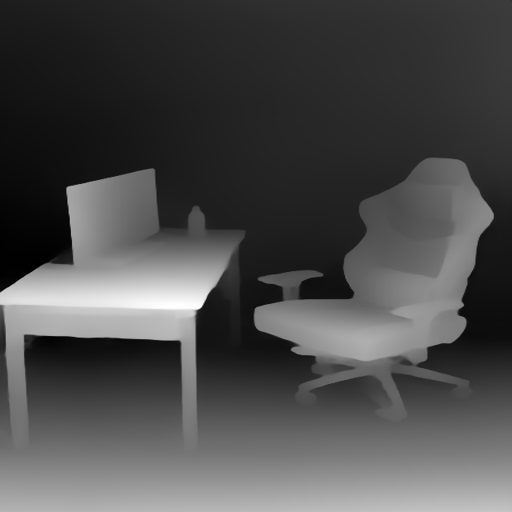 | ||
|
||
|
||
`MultiAdapter` combines keypose and depth adapters. | ||
|
||
`adapter_conditioning_scale` balances the relative influence of the different adapters. | ||
|
||
```py | ||
from diffusers import StableDiffusionAdapterPipeline, MultiAdapter | ||
|
||
adapters = MultiAdapter( | ||
[ | ||
T2IAdapter.from_pretrained("TencentARC/t2iadapter_keypose_sd14v1"), | ||
T2IAdapter.from_pretrained("TencentARC/t2iadapter_depth_sd14v1"), | ||
] | ||
) | ||
adapters = adapters.to(torch.float16) | ||
|
||
pipe = StableDiffusionAdapterPipeline.from_pretrained( | ||
"CompVis/stable-diffusion-v1-4", | ||
torch_dtype=torch.float16, | ||
adapter=adapters, | ||
) | ||
|
||
images = pipe(prompt, cond, adapter_conditioning_scale=[0.8, 0.8]) | ||
``` | ||
|
||
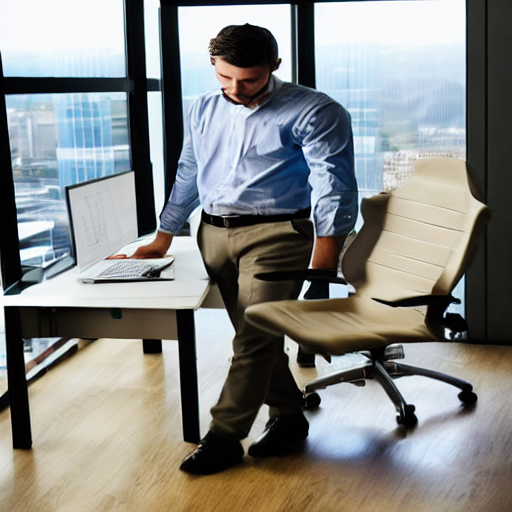 | ||
|
||
|
||
## T2I Adapter vs ControlNet | ||
|
||
T2I-Adapter is similar to [ControlNet](https://huggingface.co/docs/diffusers/main/en/api/pipelines/controlnet). | ||
T2i-Adapter uses a smaller auxiliary network which is only run once for the entire diffusion process. | ||
However, T2I-Adapter performs slightly worse than ControlNet. | ||
|
||
## StableDiffusionAdapterPipeline | ||
[[autodoc]] StableDiffusionAdapterPipeline | ||
- all | ||
- __call__ | ||
- enable_attention_slicing | ||
- disable_attention_slicing | ||
- enable_vae_slicing | ||
- disable_vae_slicing | ||
- enable_xformers_memory_efficient_attention | ||
- disable_xformers_memory_efficient_attention |
This file contains bidirectional Unicode text that may be interpreted or compiled differently than what appears below. To review, open the file in an editor that reveals hidden Unicode characters.
Learn more about bidirectional Unicode characters
This file contains bidirectional Unicode text that may be interpreted or compiled differently than what appears below. To review, open the file in an editor that reveals hidden Unicode characters.
Learn more about bidirectional Unicode characters
Oops, something went wrong.