forked from teddylee777/machine-learning
-
Notifications
You must be signed in to change notification settings - Fork 0
Commit
This commit does not belong to any branch on this repository, and may belong to a fork outside of the repository.
- Loading branch information
1 parent
e5cd9eb
commit ac0a891
Showing
1 changed file
with
297 additions
and
0 deletions.
There are no files selected for viewing
This file contains bidirectional Unicode text that may be interpreted or compiled differently than what appears below. To review, open the file in an editor that reveals hidden Unicode characters.
Learn more about bidirectional Unicode characters
Original file line number | Diff line number | Diff line change |
---|---|---|
@@ -0,0 +1,297 @@ | ||
{ | ||
"cells": [ | ||
{ | ||
"cell_type": "markdown", | ||
"id": "2ed64781", | ||
"metadata": {}, | ||
"source": [ | ||
"## GoogLeNet의 Inception Module 구현\n", | ||
"\n", | ||
"Going Deeper with Convolutions(2015) Inception 모듈에 대한 내용입니다. 해당 논문에서는 Inception Module이라는 새로운 neural network architecture 를 공개하였습니다. 논문의 제목과 같이 Going Deeper 즉 더욱 깊은 신경망 모델을 dimension reduction이 적용된 Inception Module로 가능케 하였는데, 이때 1x1 Convolution을 적극 활용하였습니다.\n", | ||
"\n", | ||
"이때 활용한 1x1 Convolution이 어떤 역할을 하였는지 살펴보도록 하겠습니다.\n", | ||
"\n", | ||
"- 논문 링크 [**(링크)**](https://arxiv.org/pdf/1409.4842v1.pdf)" | ||
] | ||
}, | ||
{ | ||
"cell_type": "code", | ||
"execution_count": 1, | ||
"id": "25cb458b", | ||
"metadata": {}, | ||
"outputs": [], | ||
"source": [ | ||
"import torch\n", | ||
"import torch.nn as nn\n", | ||
"import torchsummary" | ||
] | ||
}, | ||
{ | ||
"cell_type": "markdown", | ||
"id": "eb99f57d", | ||
"metadata": {}, | ||
"source": [ | ||
"> GoogLeNet Inception Module naive version (Version 1)\n", | ||
"\n", | ||
"" | ||
] | ||
}, | ||
{ | ||
"cell_type": "code", | ||
"execution_count": 2, | ||
"id": "de629de7", | ||
"metadata": {}, | ||
"outputs": [], | ||
"source": [ | ||
"class BaseConv2D(nn.Module):\n", | ||
" def __init__(self, in_channels, out_channels, **kwargs):\n", | ||
" super(BaseConv2D, self).__init__()\n", | ||
" self.conv = nn.Conv2d(in_channels=in_channels, out_channels=out_channels, **kwargs)\n", | ||
" self.relu = nn.ReLU(inplace=True)\n", | ||
" \n", | ||
" def forward(self, x):\n", | ||
" return self.relu(self.conv(x))\n", | ||
"\n", | ||
" \n", | ||
"class InceptionModuleV1(nn.Module):\n", | ||
" def __init__(self, in_channels, out_1x1, out_3x3, out_5x5, pool):\n", | ||
" super(InceptionModuleV1, self).__init__()\n", | ||
" self.conv1x1 = BaseConv2D(in_channels, out_1x1, kernel_size=1)\n", | ||
" self.conv3x3 = BaseConv2D(in_channels, out_3x3, kernel_size=3, padding='same')\n", | ||
" self.conv5x5 = BaseConv2D(in_channels, out_5x5, kernel_size=5, padding='same')\n", | ||
" self.pool = nn.Sequential(\n", | ||
" nn.MaxPool2d(kernel_size=3, stride=1, padding=1), \n", | ||
" BaseConv2D(in_channels, pool, kernel_size=1, padding='same')\n", | ||
" )\n", | ||
" \n", | ||
" def forward(self, x):\n", | ||
" x1 = self.conv1x1(x)\n", | ||
" x2 = self.conv3x3(x)\n", | ||
" x3 = self.conv5x5(x)\n", | ||
" x4 = self.pool(x)\n", | ||
" return torch.cat([x1, x2, x3, x4], 1)" | ||
] | ||
}, | ||
{ | ||
"cell_type": "code", | ||
"execution_count": 3, | ||
"id": "bcb38488", | ||
"metadata": {}, | ||
"outputs": [], | ||
"source": [ | ||
"inception_module_V1 = InceptionModuleV1(192, 64, 128, 32, 32)" | ||
] | ||
}, | ||
{ | ||
"cell_type": "code", | ||
"execution_count": 6, | ||
"id": "3c26aeb7", | ||
"metadata": {}, | ||
"outputs": [ | ||
{ | ||
"name": "stdout", | ||
"output_type": "stream", | ||
"text": [ | ||
"----------------------------------------------------------------\n", | ||
" Layer (type) Output Shape Param #\n", | ||
"================================================================\n", | ||
" Conv2d-1 [-1, 64, 28, 28] 12,352\n", | ||
" ReLU-2 [-1, 64, 28, 28] 0\n", | ||
" BaseConv2D-3 [-1, 64, 28, 28] 0\n", | ||
" Conv2d-4 [-1, 128, 28, 28] 221,312\n", | ||
" ReLU-5 [-1, 128, 28, 28] 0\n", | ||
" BaseConv2D-6 [-1, 128, 28, 28] 0\n", | ||
" Conv2d-7 [-1, 32, 28, 28] 153,632\n", | ||
" ReLU-8 [-1, 32, 28, 28] 0\n", | ||
" BaseConv2D-9 [-1, 32, 28, 28] 0\n", | ||
" MaxPool2d-10 [-1, 192, 28, 28] 0\n", | ||
" Conv2d-11 [-1, 32, 28, 28] 6,176\n", | ||
" ReLU-12 [-1, 32, 28, 28] 0\n", | ||
" BaseConv2D-13 [-1, 32, 28, 28] 0\n", | ||
"================================================================\n", | ||
"Total params: 393,472\n", | ||
"Trainable params: 393,472\n", | ||
"Non-trainable params: 0\n", | ||
"----------------------------------------------------------------\n", | ||
"Input size (MB): 0.57\n", | ||
"Forward/backward pass size (MB): 5.74\n", | ||
"Params size (MB): 1.50\n", | ||
"Estimated Total Size (MB): 7.82\n", | ||
"----------------------------------------------------------------\n" | ||
] | ||
} | ||
], | ||
"source": [ | ||
"torchsummary.summary(inception_module_V1, input_size=(192, 28, 28), device='cpu')" | ||
] | ||
}, | ||
{ | ||
"cell_type": "markdown", | ||
"id": "fa465dd9", | ||
"metadata": {}, | ||
"source": [ | ||
"> Inception Module with dimension reductions (Version 2)\n", | ||
"\n", | ||
"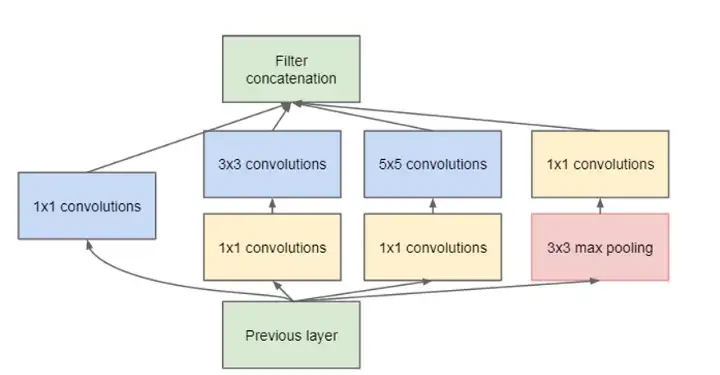\n", | ||
"\n", | ||
"출처: https://valentinaalto.medium.com/understanding-the-inception-module-in-googlenet-2e1b7c406106" | ||
] | ||
}, | ||
{ | ||
"cell_type": "code", | ||
"execution_count": 4, | ||
"id": "e787e354", | ||
"metadata": {}, | ||
"outputs": [], | ||
"source": [ | ||
"class InceptionModuleV2(nn.Module):\n", | ||
" def __init__(self, in_channels, out_1x1, out_3x3_reduce, out_3x3, out_5x5_reduce, out_5x5, pool):\n", | ||
" super(InceptionModuleV2, self).__init__()\n", | ||
" self.conv1x1 = BaseConv2D(in_channels, out_1x1, kernel_size=1)\n", | ||
" \n", | ||
" self.conv3x3 = nn.Sequential(\n", | ||
" BaseConv2D(in_channels, out_3x3_reduce, kernel_size=1),\n", | ||
" BaseConv2D(out_3x3_reduce, out_3x3, kernel_size=3, padding='same')\n", | ||
" )\n", | ||
" self.conv5x5 = nn.Sequential(\n", | ||
" BaseConv2D(in_channels, out_5x5_reduce, kernel_size=1),\n", | ||
" BaseConv2D(out_5x5_reduce, out_5x5, kernel_size=5, padding='same')\n", | ||
" )\n", | ||
" \n", | ||
" self.pool = nn.Sequential(\n", | ||
" nn.MaxPool2d(kernel_size=3, stride=1, padding=1), \n", | ||
" BaseConv2D(in_channels, pool, kernel_size=1, padding='same')\n", | ||
" )\n", | ||
" \n", | ||
" def forward(self, x):\n", | ||
" x1 = self.conv1x1(x)\n", | ||
" x2 = self.conv3x3(x)\n", | ||
" x3 = self.conv5x5(x)\n", | ||
" x4 = self.pool(x)\n", | ||
" return torch.cat([x1, x2, x3, x4], 1)" | ||
] | ||
}, | ||
{ | ||
"cell_type": "code", | ||
"execution_count": 5, | ||
"id": "76df8628", | ||
"metadata": {}, | ||
"outputs": [], | ||
"source": [ | ||
"inception_module_V2 = InceptionModuleV2(192, 64, 96, 128, 16, 32, 32)" | ||
] | ||
}, | ||
{ | ||
"cell_type": "code", | ||
"execution_count": 7, | ||
"id": "90182879", | ||
"metadata": {}, | ||
"outputs": [ | ||
{ | ||
"name": "stdout", | ||
"output_type": "stream", | ||
"text": [ | ||
"----------------------------------------------------------------\n", | ||
" Layer (type) Output Shape Param #\n", | ||
"================================================================\n", | ||
" Conv2d-1 [-1, 64, 28, 28] 12,352\n", | ||
" ReLU-2 [-1, 64, 28, 28] 0\n", | ||
" BaseConv2D-3 [-1, 64, 28, 28] 0\n", | ||
" Conv2d-4 [-1, 96, 28, 28] 18,528\n", | ||
" ReLU-5 [-1, 96, 28, 28] 0\n", | ||
" BaseConv2D-6 [-1, 96, 28, 28] 0\n", | ||
" Conv2d-7 [-1, 128, 28, 28] 110,720\n", | ||
" ReLU-8 [-1, 128, 28, 28] 0\n", | ||
" BaseConv2D-9 [-1, 128, 28, 28] 0\n", | ||
" Conv2d-10 [-1, 16, 28, 28] 3,088\n", | ||
" ReLU-11 [-1, 16, 28, 28] 0\n", | ||
" BaseConv2D-12 [-1, 16, 28, 28] 0\n", | ||
" Conv2d-13 [-1, 32, 28, 28] 12,832\n", | ||
" ReLU-14 [-1, 32, 28, 28] 0\n", | ||
" BaseConv2D-15 [-1, 32, 28, 28] 0\n", | ||
" MaxPool2d-16 [-1, 192, 28, 28] 0\n", | ||
" Conv2d-17 [-1, 32, 28, 28] 6,176\n", | ||
" ReLU-18 [-1, 32, 28, 28] 0\n", | ||
" BaseConv2D-19 [-1, 32, 28, 28] 0\n", | ||
"================================================================\n", | ||
"Total params: 163,696\n", | ||
"Trainable params: 163,696\n", | ||
"Non-trainable params: 0\n", | ||
"----------------------------------------------------------------\n", | ||
"Input size (MB): 0.57\n", | ||
"Forward/backward pass size (MB): 7.75\n", | ||
"Params size (MB): 0.62\n", | ||
"Estimated Total Size (MB): 8.95\n", | ||
"----------------------------------------------------------------\n" | ||
] | ||
} | ||
], | ||
"source": [ | ||
"torchsummary.summary(inception_module_V2, input_size=(192, 28, 28), device='cpu')" | ||
] | ||
}, | ||
{ | ||
"cell_type": "code", | ||
"execution_count": 8, | ||
"id": "30826d29", | ||
"metadata": {}, | ||
"outputs": [], | ||
"source": [ | ||
"dummy_input = torch.randn(size=(1, 192, 28, 28))" | ||
] | ||
}, | ||
{ | ||
"cell_type": "code", | ||
"execution_count": 9, | ||
"id": "5dfe3cf8", | ||
"metadata": {}, | ||
"outputs": [], | ||
"source": [ | ||
"y1 = inception_module_V1(dummy_input)\n", | ||
"y2 = inception_module_V2(dummy_input)" | ||
] | ||
}, | ||
{ | ||
"cell_type": "code", | ||
"execution_count": 10, | ||
"id": "ac2fcec2", | ||
"metadata": {}, | ||
"outputs": [ | ||
{ | ||
"data": { | ||
"text/plain": [ | ||
"(torch.Size([1, 256, 28, 28]), torch.Size([1, 256, 28, 28]))" | ||
] | ||
}, | ||
"execution_count": 10, | ||
"metadata": {}, | ||
"output_type": "execute_result" | ||
} | ||
], | ||
"source": [ | ||
"y1.shape, y2.shape" | ||
] | ||
} | ||
], | ||
"metadata": { | ||
"kernelspec": { | ||
"display_name": "Python 3 (ipykernel)", | ||
"language": "python", | ||
"name": "python3" | ||
}, | ||
"language_info": { | ||
"codemirror_mode": { | ||
"name": "ipython", | ||
"version": 3 | ||
}, | ||
"file_extension": ".py", | ||
"mimetype": "text/x-python", | ||
"name": "python", | ||
"nbconvert_exporter": "python", | ||
"pygments_lexer": "ipython3", | ||
"version": "3.7.13" | ||
} | ||
}, | ||
"nbformat": 4, | ||
"nbformat_minor": 5 | ||
} |